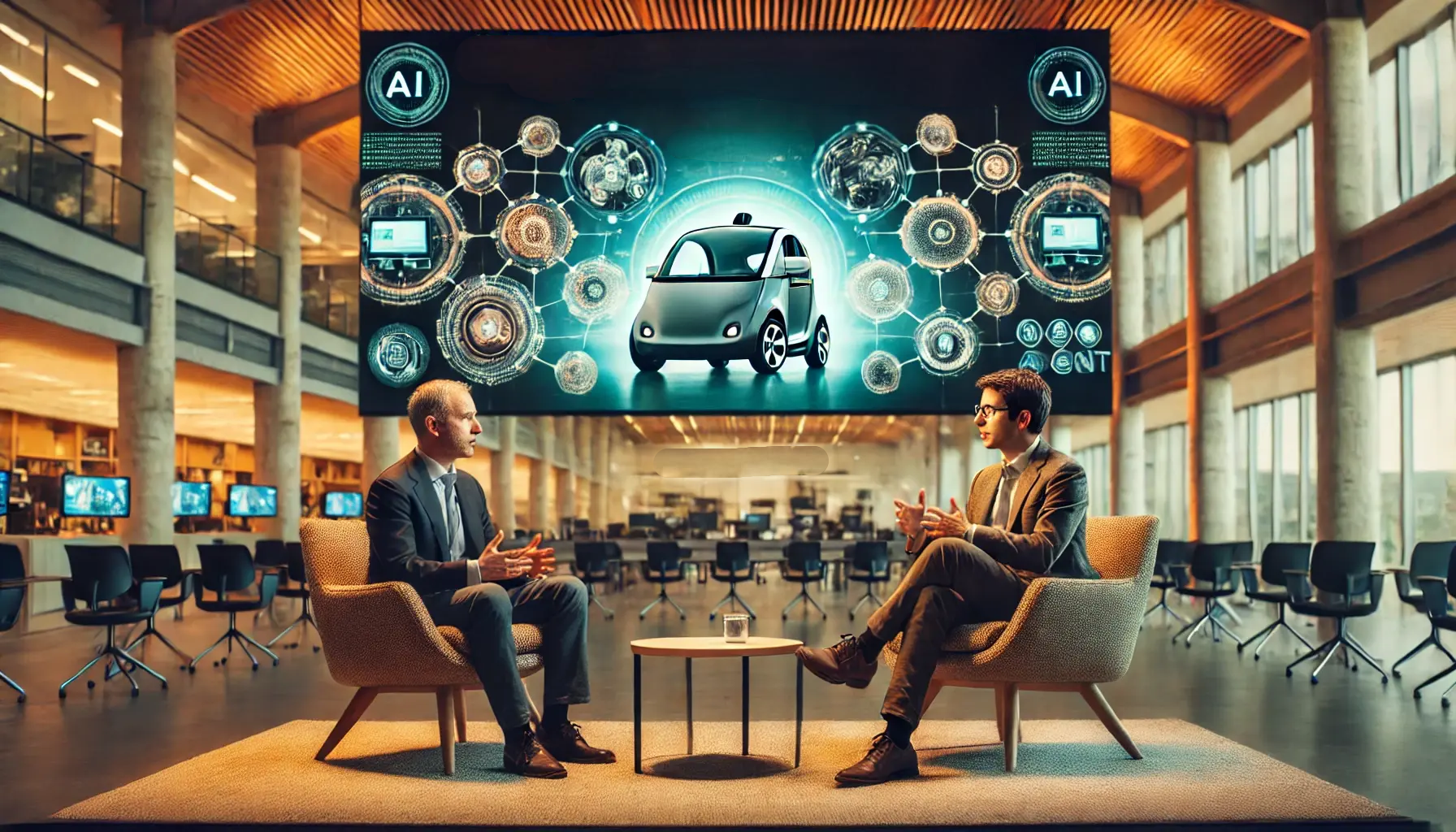
A few nights ago, we were lucky enough to host a conversation with Dr. Marco Pavone—professor at Stanford Aeronautics & Astronautics, director of Stanford’s Autonomous Systems Lab and Center for Automotive Research, head of autonomous vehicle R&D at Nvidia, and my occasional tennis buddy at Stanford.
It was the inaugural session in Akkadian Ventures’ RAISE “Sashimi Sessions,” a monthly gathering that serves fresh perspectives on emerging tech and AI. Below is a recap of our conversation, including questions from founders, GPs, and LPs: why advanced driver assistance systems (ADAS) might soon overshadow our old concept of “self-driving cars,” how generative AI is breathing new life into simulations, and how quickly we might (or might not) get from humanoid robots on stage to actual help in our homes.
Setting the Table: AVs, Agents, and Accelerating Innovation
We kicked off the night with a simple premise: Over the last decade, autonomous vehicles (AVs) have captured the public’s imagination. Now we’re seeing that same fervor shift toward “autonomous agents.” Will the breakthroughs that made robotaxis possible soon make chatbots come alive in physical form?
Marco’s immediate reaction? Let’s flip the premise. He suggests we think about it as going “from autonomous agents to autonomous vehicles.” Despite the excitement around ChatGPT and generative AI, the real brass tacks for AI revolve around moving things in the physical world—cars, trucks, drones, and robots. Whether it’s weaving through city traffic or making sense of an unpredictable environment, “physical AI” is an order of magnitude harder than (purely) digital AI.
Why? Because with physical AI, you have to layer in mechanical complexity, real-time human interaction, and absolute safety requirements.
The Simulation Superpower
No surprise: training an AV to handle every possible real-world scenario is daunting, if not impossible. We rarely record data for rarer-than-rare corner cases—like that brand-new stop sign plastered onto a highway billboard.
But new generative AI techniques can simulate hyper-realistic scenarios. Marco highlighted how large language models and video generation models can dream up road events that rarely (or never!) happen in real life, then feed them into a simulator:
- Prompt the AI with accident reports or oddball scenarios, even if it’s just a short police statement.
- Generate a realistic 3D or video representation of that scenario—complete with pedestrians, cyclists, weather effects, and even that weird stop-sign billboard.
- Train and test your AV software against the scenario, as if it were out on the street.
It’s the AV version of practice makes perfect.
We see you, Tesla ghost brakes. Marco gave the example of a Tesla that slams on its brakes for a billboard that—unfortunately—features a photo of a stop sign. With the new wave of vision-language foundation models, the software understands this is just a picture of a stop sign, and keep calm and carry on.
Do We Really Need Cars with LIDAR—Or Humanoid Robots?
Naturally, people asked about LiDAR:
- Is it essential for truly driverless cars (Level 5)?
- Or can we just rely on cameras, like Tesla’s approach?
Marco explained that for consumer-grade ADAS (think: everyday folks, not fleets), LiDAR is too pricey. Plus, cameras (especially with modern, large-scale model training) do a solid job identifying and classifying objects. For robotaxis—like Waymo—LiDAR still plays an important role because it provides redundancy (no human driver to step in if something goes sideways), and the costs are amortized differently with a vehicle that drives (and earns) all day long. But if the cost of LiDAR eventually plummets, who knows?
Then we segued into humanoid robots though Marco was quick to point out that:
- Humanoid robots face major hurdles in mechatronics and safe mechanical design.
- Autonomous cars have a simpler physics problem (four wheels vs. two legs and two arms with numerous degrees of freedom).
- Truly general-purpose home robots remain a “North Star.” Possibly a decade or more away.
Instead, the real near-term payoff might be in narrower, specialized robotics. For example, exoskeletons for mobility assistance, or highly specialized pick-and-place robots, or even “smart” fridges and home appliances that handle straightforward tasks. (Though if you’re praying for a robot that does your dishes tomorrow, maybe don’t hold your breath.)
2025: The Big Year?
Some analysts are pegging 2025 as the tipping point for mainstream robotaxis and meaningful humanoid robot prototypes. But if we’ve learned anything from the last decade, it’s that the final 10% (or sometimes 0.5%) of technical challenges can take 90% of the time.
That said, Dr. Pavone shared a sense of real optimism for the next couple of years:
- ADAS will keep getting better. And cheaper.
- Physical AI breakthroughs—especially for simulation—are piling up.
- At-scale data ingestion and training loops are self-reinforcing. The more real-world miles you collect, the better your generative model gets. The better your generative model, the more you can fill gaps with synthetic data.
Put simply, the hype is real. We may not be summoning fully humanoid butlers soon, but we’ll definitely see safer roads and advanced robotics beyond well-funded R&D labs.
Rapid-Fire Q&A
Q: If specialized robotics are closer to reality than all-purpose humanoids, why do some big players keep building scary bipedal bots?
Marco: Those efforts are more like a “North Star.” Pushing the hardest problem forces breakthroughs in smaller subsystems—like better manipulation and new sensor types. Often, that tech cascades back into simpler, narrower-use robotics that are easier to commercialize.
Q: Will we see a major scaling limit on today’s models? Do we need completely new approaches for Level 5 autonomy?
Marco: We’ll definitely hit diminishing returns on purely throwing more data and compute at the problem. However, we’re building complementary methods—like robust guardrails and advanced simulation—that supplement these big models. The synergy is what makes genuine Level 5 autonomy plausible.
Q: Any final guess on cost? Will fully autonomous cars be an extra $50K or $5K on top of a base vehicle?
Marco: Right now, advanced ADAS is maybe a few thousand in hardware + software. But truly removing the driver requires a fully redundant system. That’s still expensive, and ironically might make more sense at scale (robo-fleets) than for personal vehicles.
So, Where Does This Leave Founders?
At Near Horizon, we love “used-to-be-impossible” technology. Whether you’re working on specialized logistics robots, refining a computer vision stack for next-gen ADAS, or building tools for synthetic training data, we want to hear from you.
- The big lesson: no matter how advanced your AI is, breakthroughs happen when you combine real-world iteration with robust simulation. And thanks to generative AI, that simulation piece looks more promising than ever.
- We also see the business side: if you focus on specialized robots or targeted ADAS solutions, you might reach profitability (and big impact) sooner than if you chase the general-purpose “does everything” dream.
We see you—and we can’t wait to see what you build next.
Final Thoughts
As Dr. Pavone said, the leap from agentic AI to physical AI is huge. But so was the gap between texting your friend a cat GIF and having an LLM generate a photorealistic cat singing “Bohemian Rhapsody.” The pace is only picking up, and we’re beyond excited to help founders ride this wave responsibly and profitably.
Got a startup idea at the intersection of hardware, autonomy, and advanced AI?
Let’s talk.